Mastering Cache Management in Memoization for JavaScript Apps
Written on
Understanding Cache Management in Memoization
In our previous discussion on automatic memoization, we now turn our attention to an essential element of the process: managing the cache effectively. While memoization boosts performance by storing function outputs, unrestricted cache growth can lead to excessive memory usage. By implementing cache size limits, we can strike a better balance between speed and resource allocation.
Before diving deeper into the specifics of controlling memory use through cache size limitations, it’s beneficial to revisit the fundamental concept of memoization. Essentially, memoization is an optimization technique that saves the outcomes of costly function calls and retrieves these cached results when the same inputs are presented again. This significantly minimizes redundant calculations, thus enhancing performance across various scenarios. For a more thorough understanding of memoization and its foundational elements, I recommend reading our article, “Unlocking Efficiency in Code: The Power of Memoization.” It provides a solid base for the advanced techniques we'll be discussing, highlighting the importance of memoization in crafting efficient and high-performing code.
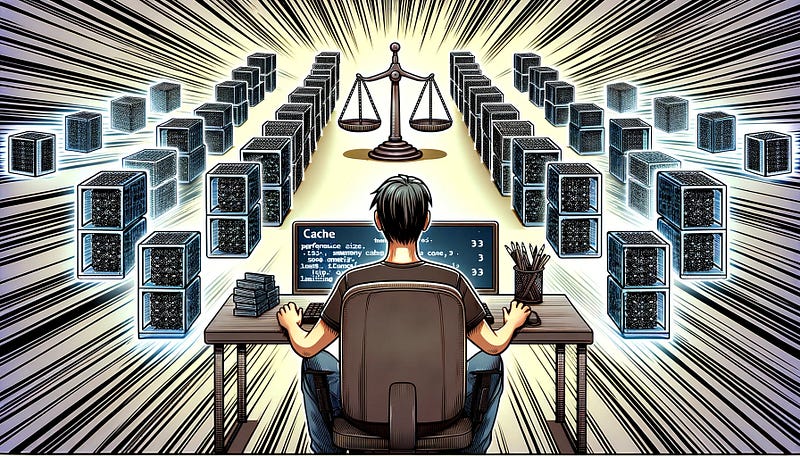
Why Implement Cache Size Limits?
Establishing limits on cache size is vital for several reasons:
- Memory Efficiency: Prevents excessive memory consumption by the memoization cache, which is particularly crucial in server-side environments where memory is a shared commodity.
- Garbage Collection: Lessens the burden on the garbage collector by allowing for better control over the lifespan of cached entries.
- Performance: Interestingly, a smaller cache can sometimes enhance performance by ensuring that only the most frequently accessed results are retained, thereby reducing lookup times.
Implementing Cache Size Limits
To set a cap on cache size, we must choose an eviction strategy—such as LRU (Least Recently Used) or FIFO (First In, First Out)—that best suits the application's requirements. In this instance, we will utilize the LRU strategy, which effectively retains the most pertinent data within the cache.
Step 1: Selecting a Suitable Data Structure
A crucial aspect of establishing an LRU cache is selecting an appropriate data structure. In JavaScript and TypeScript, a Map object is ideal due to its property of maintaining insertion order, which aligns well with the LRU principle.
Step 2: LRU Cache Logic Implementation
Below is a basic illustration of an LRU cache with a defined size limit for memoization in TypeScript:
class LRUCache {
private cache: Map<string, any>;
private maxSize: number;
constructor(maxSize: number) {
this.maxSize = maxSize;
this.cache = new Map();
}
get(key: string): any | undefined {
if (!this.cache.has(key)) {
return undefined;}
const value = this.cache.get(key);
// Move to end to indicate recent use
this.cache.delete(key);
this.cache.set(key, value);
return value;
}
set(key: string, value: any): void {
// Check if key exists and move it to the end
if (this.cache.has(key)) {
this.cache.delete(key);} else if (this.cache.size >= this.maxSize) {
// Remove the first (least recently used) entry
const firstKey = this.cache.keys().next().value;
this.cache.delete(firstKey);
}
this.cache.set(key, value);
}
}
This LRUCache class can be seamlessly integrated into your memoization function to limit cache size and enhance memory management.
Advanced Techniques and Considerations
- Dynamic Cache Sizing: Explore algorithms that adjust cache size based on the current load and available memory.
- Monitoring and Metrics: Implementing monitoring for cache hits, misses, and evictions can yield valuable insights into optimizing cache size and the overall effectiveness of your memoization strategy.
Setting cache size limits within memoization routines is a crucial strategy for optimizing memory usage and ensuring the scalability of applications. By adopting methods like LRU and employing intelligent cache management techniques, developers can reap the benefits of memoization without sacrificing resource efficiency.
For additional insights on application optimization, revisit our earlier articles, including “Unlocking Efficiency in Code: The Power of Memoization,” and delve into “Streamlining TypeScript Logic: Effective Refactoring of If-Else Blocks” for further best practices in code efficiency.
The first video discusses how to optimize cache memory performance and increase cache speed, providing practical insights into effective cache management.
The second video focuses on advanced optimization techniques for cache memory performance within computer architecture, offering deeper knowledge for developers.
To keep updated with more articles like this, follow me on Medium, or subscribe to receive my latest stories via email. You may also want to check out my lists or explore some of these related topics:
- Front-End Development Essentials
- Building Better Web Structures with Semantic Elements
- Integrating Lit Web Components in React: A Practical Guide with Examples
- Effective State Management in Lit Components: A Developer’s Guide